Digital Twin
At ManageCT, we are designing the next generation of clinical trials. With our new Digital Twin concept, which are virtual models of patients or processes, we can improve the efficiency and efficacy of clinical trials, while ensuring patient safety. These digital twins can predict how treatments might work, helping researchers design better trials and reduce risks. This makes drug development faster, safer, and can add more value for each patient.
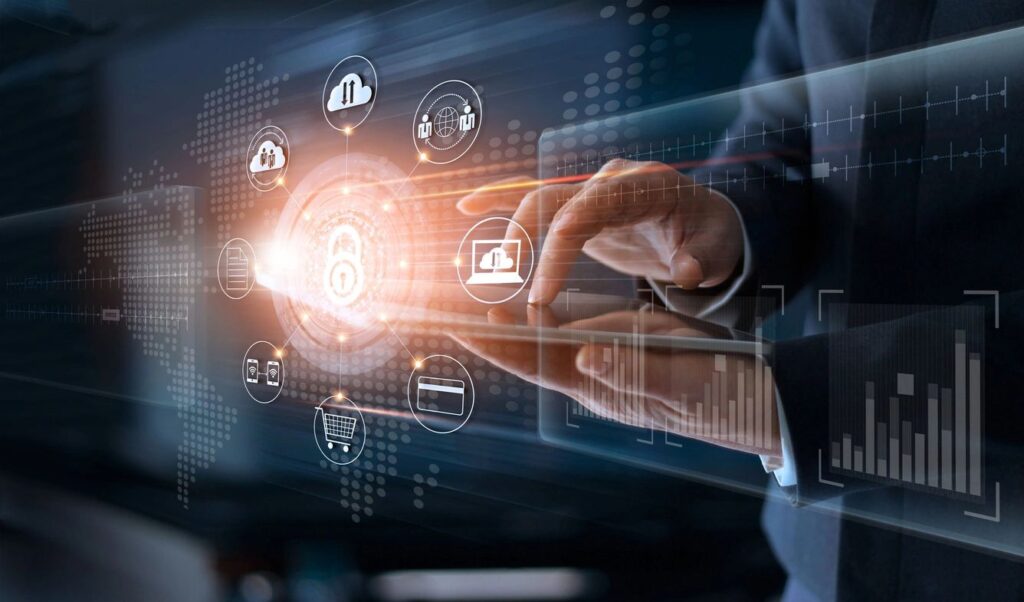
Digital Twins and AI in Clinical Trials: Revolutionizing Medical Research
A digital twin is a virtual replica of a physical system or process. In healthcare, digital twins are patient-specific models that simulate an individual’s anatomy, physiology, and disease progression, using data such as medical imaging, genomic profiles, and health records. When combined with Artificial Intelligence (AI), digital twins become powerful tools for optimizing clinical trials.
Applications in Clinical Trials
Personalized Trial Design
- Simulation of Patient Responses: Digital twins can predict how an individual might respond to a particular treatment by modeling their unique biology and disease dynamics.
- Stratification of Participants: AI-powered analysis of digital twins identifies patient subgroups most likely to benefit from a therapy, improving trial efficiency and outcomes.
Predictive Modeling
- Treatment Efficacy: AI uses digital twin data to simulate different therapeutic regimens, helping researchers identify the most promising interventions before live trials begin.
- Safety Analysis: Digital twins predict adverse events by simulating drug interactions, offering insights into potential risks for specific populations.
Optimized Recruitment
- Virtual Cohorts: AI-enabled digital twins allow researchers to create virtual cohorts, expanding the study pool and reducing the number of participants required in early trial phases.
- Recruitment Feasibility: Predictive modeling determines the availability of suitable participants, based on real-world data and digital twin profiles.
Adaptive Trial Designs
- Digital twins enable real-time updates to protocols, as simulated outcomes provide ongoing insights. AI ensures efficient recalibration of dosage, timelines, or inclusion criteria, making trials more dynamic and responsive.
Ethical and Cost-Effective Testing
- Reduced Animal and Human Trials: By simulating drug effects in digital twins, researchers minimize reliance on animals and reduce human exposure to potentially harmful treatments during early phases.
- Lower Costs: Simulations save costs by identifying ineffective compounds and optimizing trial designs before physical implementation.
Real-Time Monitoring and Predictive Analytics
- AI continuously analyzes data from digital twins during trials, predicting trends like dropout rates or unexpected responses.
- This improves trial retention and ensures early detection of anomalies.
Benefits
- Increased Success Rates: Tailoring treatments to simulated outcomes enhances the likelihood of successful trials.
- Faster Development: Simulating and refining protocols reduces the time needed for regulatory approvals and live trials.
- Reduced Risk: Predicting safety and efficacy issues lowers the likelihood of costly trial failures.
- Enhanced Diversity: Digital twins enable the inclusion of underrepresented populations in simulations, promoting more equitable results.
Challenges and Limitations
- Data Integration: Building accurate digital twins requires comprehensive, high-quality data from multiple sources, which may be fragmented or incomplete.
- Model Validation: Ensuring digital twins accurately represent real-world patients is crucial for reliable outcomes.
- Ethical Concerns: Using patient data to create digital twins raises privacy and security issues.
- Computational Demand: High-fidelity digital twins demand significant computational resources, particularly when used for large cohorts.
Case Study Example
A pharmaceutical company conducting a Phase II cancer drug trial used digital twins to predict treatment outcomes for various tumor profiles. AI analyzed patient data, suggesting optimal dosage and identifying patients at higher risk for side effects. These insights streamlined the trial process, saving time and reducing costs by 25%.
Future Directions
- Integration with Wearables: Real-time patient data from wearables will enhance the accuracy of digital twin simulations.
- Global Trial Networks: Sharing digital twin models across organizations can foster collaboration and accelerate innovation.
- Regulatory Acceptance: Standardizing digital twin methodologies will ensure broader adoption in clinical trial protocols.
Digital twins combined with AI hold the potential to transform clinical trials, making them more precise, efficient, and ethical. As technologies mature, this innovative approach will likely become a cornerstone of drug development and personalized medicine.
Get Updates And Stay Connected:
Subscribe To Our Newsletter
Why We Started ManageCT:
Why We Started ManageCT:
At ManageCT, our mission is to revolutionize clinical trials by using AI to accelerate research, enhance data accuracy, and ensure ethical practices. Our commitment to excellence and innovation drives us to optimize every trial aspect, improving patient outcomes and advancing healthcare.
Contact Information
- Phone: +1 (713) 858-9184
- [email protected]
-
51 Ruby Hill
Irvine, CA 92602